Proefschrift
Unraveling temporal processes using probabilistic graphical models
Real-life processes are characterized by dynamics involving time. Examples are walking, sleeping, disease progress in medical treatment, and events in a workflow.
- Auteur
- De Paula Bueno, M.L.
- Datum
- 11 februari 2020
- Links
- Thesis in Leiden Repository
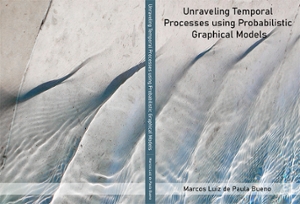
Real-life processes are characterized by dynamics involving time. Examples are walking, sleeping, disease progress in medical treatment, and events in a workflow. To understand complex behavior one needs expressive models, parsimonious enough to gain insight. Uncertainty is often fundamental for process characterization, e.g., because we sometimes can observe phenomena only partially. This makes probabilistic graphical models a suitable framework for process analysis. In this thesis, new probabilistic graphical models that offer the right balance between expressiveness and interpretability are proposed, inspired by the analysis of complex, real-world problems. We first investigate processes by introducing latent variables, which capture abstract notions from observable data (e.g., intelligence, health status). Such models often provide more accurate descriptions of processes. In medicine, such models can also reveal insight on patient treatment, such as predictive symptoms. The second viewpoint looks at processes by identifying time points in the data where the relationships between observable variables change. This provides an alternative characterization of process change. Finally, we try to better understand processes by identifying subgroups of data that deviate from the whole dataset, e.g., process workflows whose event dynamics differ from the general workflow.