Research project
Improving vegetation representation in Multi-sensor Earth Observation Products through phenology and trait-based priors
What are the behaviours of plant traits throughout various points in the growing season in a radiative transfer model framework and how well can this knowledge be integrated through data assimilation to provide priors for robust local and global vegetation products and analysis?
- Duration
- 2016 - 2020
- Contact
- Amie Corbin
- Funding
-
EU Horizon 2020
- Partners
Participant No Participant organisation name Country 1 University Leiden (UL) NL 2 University of Munich (LMU) Germany 3 University College London (UCL) UK 4 Brockmann Consultant Gmb (BC) Germany 5 Tartu Observatory (TO) Estonia 6 University of Alcala (UAH) Spain 7 Assimila UK 8 ADAS UK 9 UVSQ-LSCE France
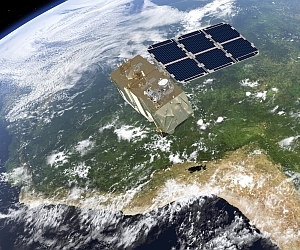
Short abstract
The main objective of this research is to better understand and define typical behaviours of plants by their functional traits throughout the growing season. However, the traits that are studied and emphasized are only those that are able to be utilized by Earth Observation satellites, such as chlorophyll, leaf water content, etc. The understanding of the phenological changes of these traits in addition to possible trait trade-offs will lead to the creation of priors (prior knowledge and models) which will be used in a data assimilation process to provide better represented in modelling, radiative transfer models (RTMs), and earth observation products which have a wide range of applications in environmental, ecological and biological research.
Photo Sentinel-2: (c) ESA/ATG medialab 2015
Project description
This project is a part of the MULTIscale SENTINEL land surface information retrieval PLatform (MULTIPLY) project which is developing a data assimilation platform for land surface products. This platform will provide land surface products at local and global scales such as chlorophyll, biodiversity, soil moisture, etc. which can be used by scientists or the industry from various fields for spatial analysis.
As a part of the schematic framework, priors are required for consistent and reliable information as a check to bring comparable and gap-free data to a variety of users. This project aims to focus on vegetation priors through RTMs, mainly focusing on functional plant traits and phenology. This will initially be conducted through large meta-analysis to create several priors to add to a data assimilation platform MULTIPLY in relation to several aspects of vegetation phenology.
However, many previous studies focus only on collecting traits at a peak-biomass, or the height of the growing season. In order to provide more accurate and robust observations, additional data from a variety of global sites will be collected in parallel with spectral information. This will allow us to observe the change of plant trait and reflectance values over a growing season. Once data is collected, priors will be formed by an exploration of covariance, spatial and temporal scales/ranges, hyperspectral and multi-spectral outputs, as well as predictive phenological trait models.