
John Mydosh and the mystery of the Hidden Order
A 35-year-old uranium crystal will not disclose its secret: what causes a dramatic phase transition at 17.5 Kelvin? Thanks to a new artificial intelligence approach, half of the possible explanations are excluded, but the definitive answer remains to be found. 'It is very frustrating', says physicist John Mydosh (82).
It all started so promisingly: in the eighties, solid-state physicist John Mydosh and his Leiden colleagues got their hands on a batch of uranium-containing crystals. Uranium is not only interesting for nuclear physicists, but it's also a giant atom carrying an electron cloud of 235 electrons. This could easily lead to exotic phenomena in crystals containing it.
And indeed, the compound uraniumrutheniumsilicon (URu2Si2) fulfilled the promise. 'When you cool this down to 17.5 Kelvin (degrees above absolute zero, -273 degrees Celsius), you see a clear phase transition', says John Mydosh (82) an originally American physicist who just kept doing physics after his retirement as a Leiden professor.
A phase transition is a sudden change of the physical properties of a material when a parameter is changed, such as the temperature. At 100 degrees Centigrade, water will start to cook: it changes from a liquid into a gas. Another example is magnetism in iron: below the transition temperature (770 degrees Centigrade), an iron crystal has an inherent magnetic field. Above it, the field disappears.
'We turned out to be wrong'
Mydosh and colleagues knew there was such a transition from changes in the heat capacity around 17.5 Kelvin, but what was the cause? In 1985, they published a much-cited article in Physical Review Letters, in which they explain the phase transition by antiferromagnetism, a variant of magnetism. Case closed? 'Well, we turned out to be wrong', says Mydosh.
The theory of phase transitions tells us that there has to be an order parameter. In freezing water, it is the degree to which the molecules are fixed in a regular lattice. Mydosh: 'We didn't know what the order parameter was in this case. It wasn't magnetism, no changes in the crystal, no superconduction. We just don't know. That is why we call it hidden order'
Adding intrigue is the fact that URu2Si2 shows another phase transition at 1.5 Kelvin, this time to a superconducting state, one of the large mysteries in physics. Both phase transitions seem to have a connection: 'When you push away the hidden order transition, for example by applying pressure, the superconducting transition also disappears', explains Mydosh.

Frustration
More than a thousand scientific papers about the problem have appeared since then, in which scores of theories have been launched. However, none have been accepted by the physics community, says Mydosh. 'Two years ago, when I was still young, we were at the synchrotron in Hamburg for two weeks of measurements with a hypothesis for hidden order. That is heavy duty work, day and night. But well, we couldn’t verify it, so nothing came out of these efforts and we never published our negative result.'
'A big frustration', says Mydosh about the unsolved mystery, even though there were plenty of successes in his career. Mydosh is the man behind spin glasses, a phenomenon that he wrote a much-cited book about in the 1990s. And fellow solid-state physicists may know him from his work on Colossal Magnetoresistance, a physical effect that is used in disk drives.
In the meantime, his co-authors are emeriti or have moved on to other things, but Mydosh still publishes almost yearly, mostly about the uranium mystery. 'In 2015 I had a good one in Science. Why didn't you write about that then?', he asks.
Now, there is an article in Science Advances, describing a neural network, a form of artificial intelligence. 'It's in fashion, artificial intelligence is hip hop', jokes Mydosh, who is far from an expert himself, although coauthor Eun-Ah Kim of Cornell University in New York is.
The researchers use Resonant Ultrasound Spectroscopy (RUS), a new experimental technique where the crystal is inserted in a cavity between two ultrasound elements. The first elements send ultrasound, with frequencies hundreds of thousands of vibrations per second into the crystal. The second element detects the strength of the resonances that make it through the crystal.
The whole process can be compared to knocking a material and listening to the sound. Metal yields a clear 'ping', wood is more of a 'tock', and glass has yet another different sound. Each crystal has a number of resonance frequencies, determined by the elastic constants of the crystal, the way the atoms vibrate in the crystal lattice due to the forces between those atoms. By tracing the resonances while the temperature varies, the phase transition becomes visible. Some resonances show just a change of direction there, a simple u-turn, while other resonances form a double turn, more of an s-shape.
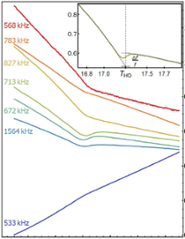
U- and S-turns
Those u-turns and s-shapes tell us something about the mysterious phase transition. Theories about that can be divided into two main categories: theories in which the hidden order parameter is a single number, and theories where it is two-dimensional. In the last case, more resonance curves should have s-turns than when the order parameter is one number.
A practical problem is that the largest crystals, which have the clearest signal and a nice, polished form, tend to have more defects in the crystal structure, which can obscure the resonance spectrum. Smaller crystals have a better crystal structure, but the signal is not as strong, and it's more troublesome to polish them in appropriate shape for a good RUS-analysis.
In both cases, this makes interpreting the resonance spectrum harder. One missing or unclear resonance frequency can spoil the whole analysis. For this reason, the translation from u-turns and s-shapes back to an order parameter is very difficult. Here machine learning can be helpful.
Artificial Neural Network
A computer can easily simulate a crystal, including shape, temperature and a one- or two-component order parameter. While the temperature varies, the model calculates the shape of the detected resonance frequencies.
To make use of this, the researchers used an artificial neural network, a type of artificial intelligence loosely based on the way brain cells or neurons work. To recognize patterns, neural networks have to be trained, like athletes or pets. The ANN simulated spectrum goes into one end of the network, and on the other side, an answer spits out: either the order parameter has one-component or two components.
When the answer is correct, the connections between the neurons are enhanced, if the answer is wrong, they are adapted. By repeating this thousands of times, using different simulated crystals, the network learns to recognize the patterns that point to one or two-component order parameters. They increased the robustness of that pattern recognition by omitting random resonance frequencies from the training spectra. This way, the network is prepared for non-perfect measurements with missing frequencies.
Training
A computer can easily simulate a crystal, including shape, temperature and a one- or two-component order parameter. While the temperature varies, the model calculates the shape of the detected resonance frequencies.
To make use of this, the researchers used an artificial neural network, a type of artificial intelligence loosely based on the way brain cells or neurons work. To recognize patterns, neural networks have to be trained, like athletes or pets. The network simulated spectrum goes into one end of the network, and on the other side, an answer is spit out: either the order parameter has one-component or two components.
When the answer is correct, the connections between the neurons are enhanced, if the answer is wrong, they are adapted. By repeating this thousands of times, using different simulated crystals, the network learns to recognize the patterns that point to one or two-component order parameters. They increased the robustness of that pattern recognition by omitting random resonance frequencies from the training spectra. This way, the network is prepared for non-perfect measurements with missing frequencies.
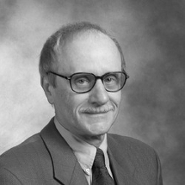
The mystery remains
When the network, after thousands of training rounds, had finally learned to determine the order parameters of simulated crystals, the researchers fed it some real experimental measurements. It worked: the network clearly indicated that these were spectra with a one-component phase transition.
'It turns out that the patterns are clearly separable', says Mydosh. From twenty candidate theories of hidden order, this eliminates about half, so now there remain about ten to test further.' That’s nice, even if it doesn't solve the mystery by a far stretch.
But, the authors write, this approach can be used more widely. 'Any technique where simulation of a dataset is straightforward but where fitting is difficult should be amenable to a framework of the type used here', they write.
In the meanwhile, John Mydosh will search for the definitive theory.