Research project
CIMPLO – Maintenance prediction for industries
Researchers of the Leiden Institute of Advanced Computer Science (LIACS) have started a 4 year project on developing a system that sends out automatic alerts when components from engines are showing first signs of fatigue.
- Duration
- 2017 - 2021
- Contact
- Thomas Bäck
- Funding
-
Technology foundation NWO-STW
- Partners
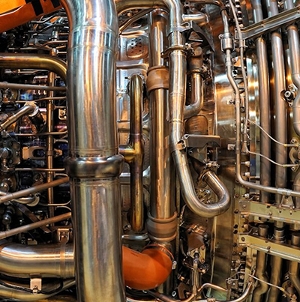
Perfect moment
Together with KLM, Honda and CWI, thereby supported by Damen, Tata Steel, DAF Trucks and the University of Amsterdam, new optimization algorithms will be developed into a cross industry, big data platform. This platform aims at optimizing the perfect moment (both cost-wise and safety-wise) to perform maintenance on engines.
Aim
The CIMPLO-project aims at developing a cross-industry predictive maintenance optimization platform, which addresses the real-world requirements for dynamic, scalable multiple-criteria maintenance scheduling. To achieve the full business advantages in terms of safety, time and financial savings, the CIMPLO-project combines predictive maintenance with dynamic multi-objective scheduling, such that maintenance events and the required assets can be dynamically (re-)scheduled.
Critical activity
Every enterprise has to deal with it: maintenance of machinery and infrastructure. Traditional maintenance concepts rely on a ‘fixed interval approach’, which takes into account a significant safety margin. As a consequence, maintenance is almost always taking place too early or, in worse cases, too late. This makes it probably one of the most inefficient, and at the same time most critical, activities in industry.
High potential of sensors
Today, all parts of industrial machines are monitored with numerous sensors. Together, they provide a massive amount of data, potentially useful for maintenance. For project partners KLM and Honda, examples of those machines are aircraft and passenger car engines, respectively. Onboard sensors provide the possibility to model the degradation of parts in both engine types. The data can be used for predictive modelling and dynamic multi-objective scheduling.
Predictive maintenance
The appropriate infrastructure for collecting, preprocessing, and analysing big data, will in turn open the door to predictive maintenance: optimize the best moment to perform maintenance. Companies will be able to schedule it at their own convenience, thereby preventing unexpected failure of their systems. The CIMPLO approach will drive down costs immediately, while preserving proper safety levels and human capital deployment.
Applicable to any industry
CIMPLO is a project of the Leiden Institute of Advanced Computer Science, Centrum voor Wiskunde en Informatica, and industrial partners KLM and Honda. Funding has been received from research fund STW. Ultimately, the platform is applicable to any industry.