Dissertation
Self-Adjusting Surrogate-Assisted Optimization Techniques for Expensive Constrained Black Box ProblemsBagheri, S.
Optimization tasks in practice have multifaceted challenges as they are often black box, subject to multiple equality and inequality constraints and expensive to evaluate.
- Author
- Bagheri, S.
- Date
- 08 April 2020
- Links
- Thesis in Leiden Repository
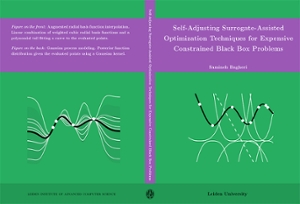
Optimization tasks in practice have multifaceted challenges as they are often black box, subject to multiple equality and inequality constraints and expensive to evaluate. The efficiency of a constrained optimizer has a crucial importance when it comes to selecting a suitable method for solving real-world optimization problems from industry with strict resource limitations. The primary concern of this work is to develop new black box optimization algorithms which are generic enough to successfully handle a broad set of constrained optimization problems (COPs) efficiently and without requiring apriori parameter tuning for different classes of the problems. To achieve this goal we benefit from two main conceptual components in the development of new constrained solvers: 1. utilizing surrogate modeling techniques to save real function evaluations, 2. automatically adjusting sensitive problem-dependent parameters based on the information gained about the problems during the optimization procedure. This work eventuated in the development of two surrogate-assisted constrained solvers: SACOBRA and SOCU. It turns out that SACOBRA outperforms most other COP-solvers in solving the well-known G-problem suite and MOPTA08 (a COP from automotive industry), if the number of function evaluations is strongly limited.