Dissertation
Bayesian Inference for Gaussian Models
This dissertation studies the Asymptotics of Bayesian nonparametric inference for Gaussian linear models.
- Author
- Yan, D.
- Date
- 03 March 2020
- Links
- Thesis in Leiden Repository
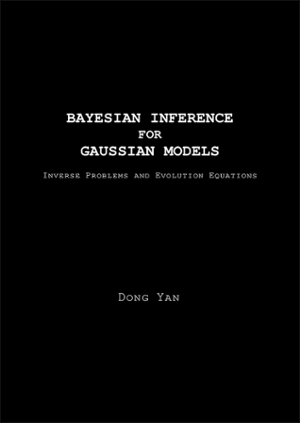
This dissertation studies the Asymptotics of Bayesian nonparametric inference for Gaussian linear models. In Part I, we collect the materials essential for the development of this thesis, including smoothness classes, the Gaussian noise model, and the general approach of Bayesian nonparametric inference to Gaussian linear models. Part II investigates linear inverse problems with Gaussian noise. We examine the inverse problems with continuous observations in smoothness scales in Chapter 6. The inverse problems with discrete observations are studied in Chapter 7 using Gaussian conjugacy and in Chapter 8 by extending the results from Chapter 6. Our results demonstrate two facts. First, the Bayesian contraction rates reach the same order of frequentist minimax optimal rates. Second, there is no deterioration in posterior contraction rates due to the information loss from continuous to discrete observations in inverse problems. Part III covers Bayesian nonparametric inference for stochastic evolution equations. We derive the contraction rates of Gaussian priors for initial conditions and drifts. The results show that the rates are affected by the signals with anisotropic smoothness in higher dimensions.